A recurring challenge in biology is the extraction of ever more spatiotemporal information from targets, as a myriad of transient cellular processes occur in three-dimensional (3D) tissues and across long timescales. Several imaging techniques, including epifluorescence and selective plane illumination microscopes, can image live samples in three dimensions at high spatial resolution. However, they require a number of two-dimensional (2D) images through scanning to create a 3D volume, thus the volume rate is compromised by the extended acquisition time by the camera. To capture the 3D dynamic events in milliseconds, such as heartbeat, blood flow, neural activity, etc., still remains challenging for most of the hardware.
To address the above-mentioned problems, the Peng Fei’s groupfromSchool ofOptical and Electronic Informationrecently published a paper entitled "Real-time volumetric reconstruction of biological dynamics with light-field microscopy and deep learning" on the well-known top journal《Nature Methods》, reportinga deep-learning based light-field imaging techniques with realizing sustained 3D imaging of fast dynamic processes. This technique combined a view-channel-depth (VCD) neural network reconstruction approach with cutting-edge light-field microscopy, yielding 3D video at superior spatiotemporal resolution with ~1 Giga voxels per second reconstruction throughput, which is over two‐order‐of‐magnitude faster than current 3D imaging implementations.
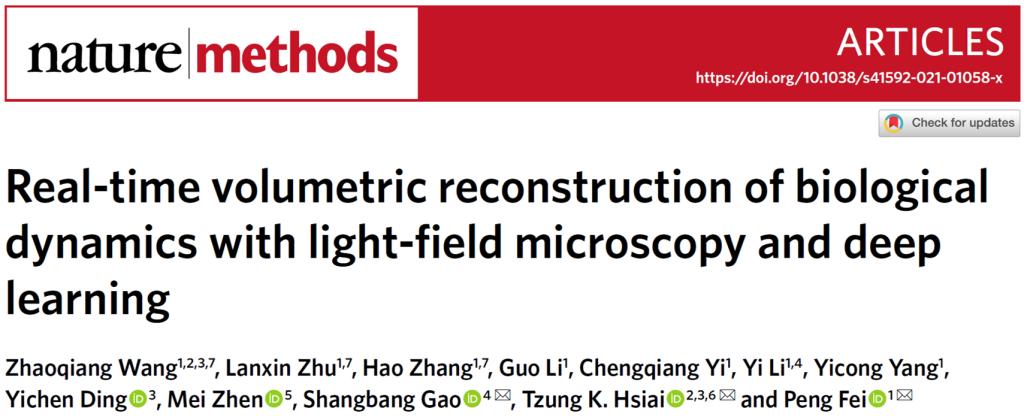
Light-field microscopy (LFM) acquires both the position and angle information (i.e. the light field) of the sample’s fluorescence in single snapshot. The VCD neural network further provides a deep learning pipeline to process the light field and reconstruct into 3D image stack in post-imaging stage. Hence it allows for an equivalent volume rate of over 100 Hz to the dynamic process. This method breaks the limit of spatial bandwidth product (optical throughput) of the current 3D imaging technology. It successfully captures the millisecond dynamic biological process of live samples with single-cell resolution, and can achieve a reconstruction throughput of 13 volumes s-1. Based on the high temporal and spatial resolution of this method, Peng Fei 's group and Gao Shangbang's group cooperated to capture the neuron calcium signaling of the fast-movingC.elegansat an acquisition rate of 100Hz and extract the four-dimensional spatiotemporal patterns of neuronal calcium signaling and track correlated worm behaviors at single-cell resolution (Figure 1a). Fei Peng's group also cooperated with Hsiai's group to demonstrate this technology in toto imaging of blood flow in the beating heart of zebrafish larvae, enabling velocity tracking of blood cells and ejection fraction analysis of the heartbeat with volumetric imaging rates up to 200 Hz (Figure 1b).
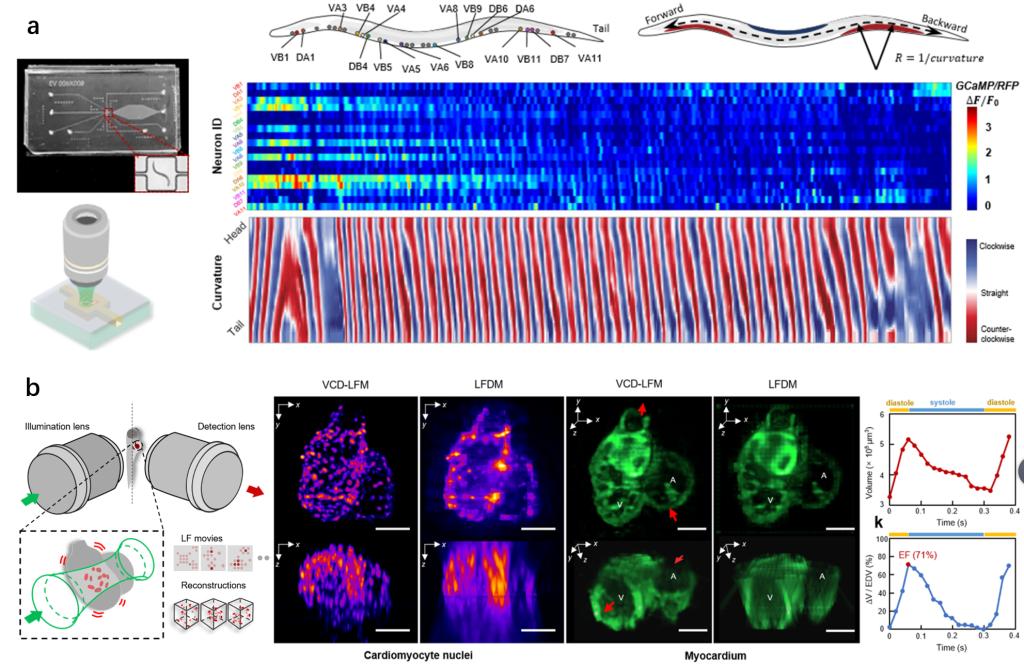
Figure 1. The deep-learning based light-field microscopy realized the in toto imaging of neuronal activities across movingC.elegansand blood flow in a beating zebrafish heart at single-cell resolution with volumetric imaging rates up to 200 Hz.
In conclusion, the research has developed a novel deep-learning based light-field microscopy that achieves real-time recording and video-rate reconstruction of instantaneous 3D processes at single cell resolution, which are difficult for current 3D microscopy. Its superior performance is demonstrated on many milisecond transient biological dynamics such as the neuronal activities of movingC.elegansand blood flow in a beating zebrafish heart.
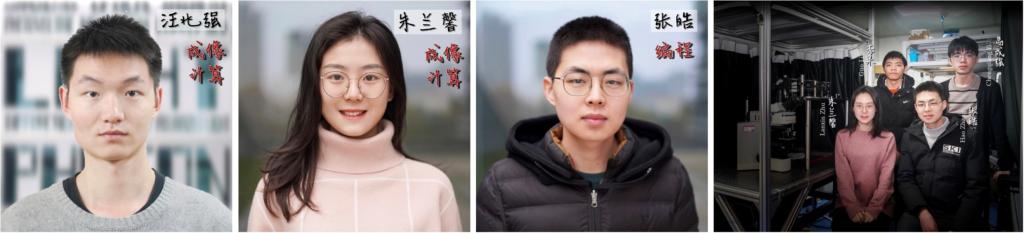
Wang Zhaoqiang (Undergraduate of 2014 at HUST, PhD student of 2018 at UCLA), Zhu Lanxin ( Phdstudent of 2018 at HUST) and Zhang Hao (Phd student of 2019 at HUST) are the co-first authors of this paper. Professor Fei Peng from the School ofOptical and Electronic Information, Professor Gao Shangbang from the College of Life Sciences and Technology, and Professor Hsiai Tzung from School of Medicine at UCLA are the co-corresponding authors of this paper. This research This work was supported by the following grants: National Natural Science Foundation of China, National Key R&D program of China, Innovation Fund of WNLO, National Science Foundation of Hubei, National Institutes of Health (NIH), Department of Veterans Affairs, the Fundamental Research Funds for the Central Universities , and the Junior Thousand Talents Program of China.
Paper link:https://rdcu.be/cgyoA